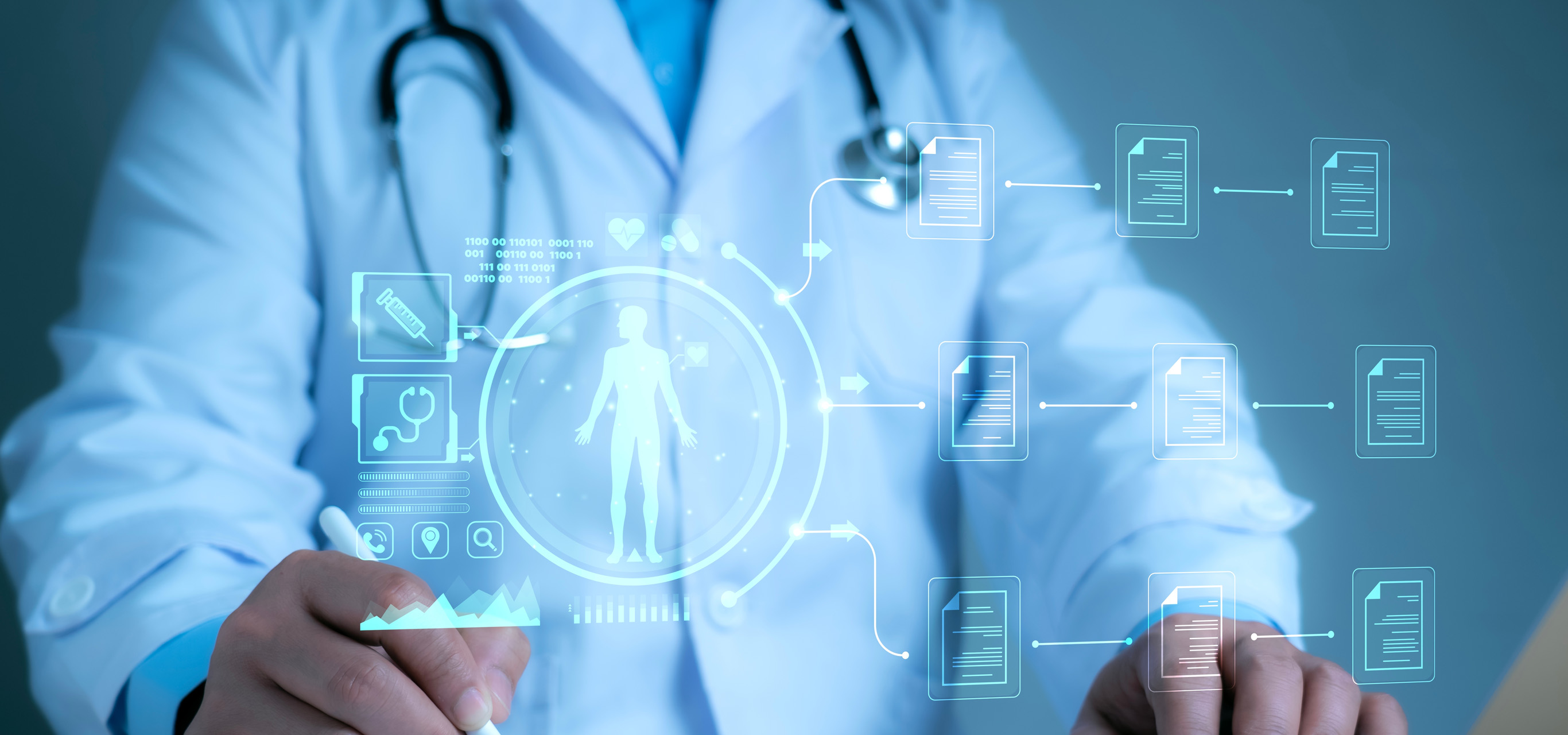
Support for Assessing Therapeutic Efficacy in Patients with Inflammatory Bowel Disease through Unstructured Data in Electronic Health Records
At NTT DATA, we recently worked with Ono Pharmaceutical Co., Ltd. on a research study analyzing unstructured electronic health records (EHRs) data from patients with inflammatory bowel disease (IBD). The study aimed to identify the characteristics of IBD patients who exhibit resistance to medications, understand real-world treatment situations, and ultimately improve care. The aim of this project is to perform morphological analysis on electronic health records texts and have text mining processes to extract keywords characteristic of treatment-resistant patients. Rather than conducting extensive analyses such as building AI models, the focus will be on these text-based approaches.
EHRs data was extracted from the Millennium Medical Records, which contained physician progress notes, clinical summaries, and other free-text information. Natural language processing (NLP) was leveraged to detect specific keywords that physicians use when documenting poor medication response in IBD patients.
The study results demonstrating the potential of this approach were shared at the Meeting of the Japan Association for Medical Informatics. By enhancing understanding of IBD patient journeys and outcomes, the researchers hope to reduce burden and enable more personalized, effective treatment.
This research serves as an initial building block for automatically detecting drug treatment resistance based on documented symptoms and creating digital biomarkers to measure medication efficacy. Moving forward, we would like to expand the dataset and refine the methodology by combining keywords and using AI technologies. The ultimate goals are to ease the burden on patients and enable patient specific, effective therapies through an enhanced understanding of real-world responses.
Background of the Study
IBD generally refers to two conditions: ulcerative colitis and Crohn's disease. The exact cause remains unknown, however both diagnoses often necessitate prolonged treatment. Although newer immunomodulating drugs have emerged, many patients demonstrate resistance, reducing the efficacy of treatment. Consequently, there is a pressing need to uncover factors contributing to medication resistance from sources like real-world clinical data.
Recently, real-world data (RWD) from clinical settings has shown promise for capturing insights around patient experiences, medication impacts, and procedure outcomes. This RWD is built from diverse sources such as insurance claims, DPC surveys , and electronic medical records.
However, much RWD in Japan consists solely of insurance claims and DPC survey results detailing medical procedures and lacks critical patient journeys and intervention results. Due to these limitations in the data sets, understanding care processes and outcomes has remained difficult.
Today's Millennium Medical Records , however, contains more in-depth physician-documented records, including progress notes. These free-text notes better reflect patient progression over time.
In this work, the focus was leveraging such narrative clinician documentation within EHRs. The ultimate goal is to develop techniques to identify patients resistant to IBD drug therapies based on symptoms and observations recorded in standard practice.
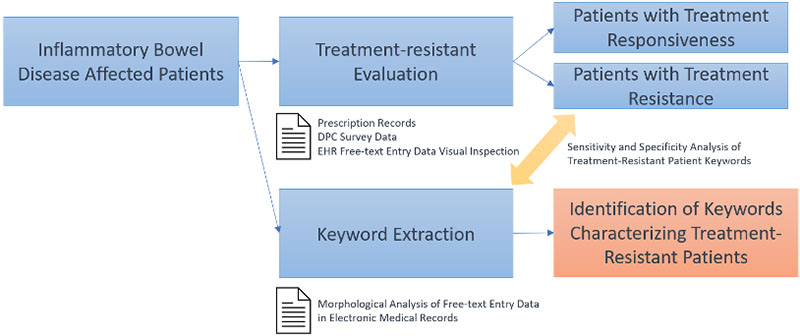
Research Objective |
The research aims to explore methodologies in extracting clinical outcomes like drug treatment resistance from the free-text data in electronic medical records of IBD patients. The study will utilize the Millennium Medical Records and apply NLP to the unstructured physician notes and summaries. This allows outcomes to be identified that typically cannot be captured through insurance claims data alone. Additionally, the research includes evaluation of the accuracy of this extraction methodology. |
---|---|
Research Period | March 2023 - July 2023 |
Research Methodology |
The sample consisted of 210 IBD patients out of 866 in the Millennium Medical Records that had treatment history with the targeted medications. Manual review of the free-text progress notes and summaries was conducted to label treatment resistance. NLP was then applied to this free-text data to identify potentially useful keywords for determining resistance. Synonym registration and differentiation of positive/negative language was utilized. Subsequently, the accuracy of these keywords in indicating resistance could be assessed. |
Roles |
Ono Pharmaceutical Co., Ltd.:
NTT Data:
|
Results of Research |
Analysis examined the ability of keywords to identify treatment resistance based on physician notes. Sensitivity and specificity levels were compared between terms. "Improvement none," "fasting," "aching pain," and "bleeding" showed relatively high sensitivity and specificity. Their presence suggests a higher probability of resistance on the other hand, their absence suggests a lower probability. These terms are considered viable candidates for detecting resistance.
Furthermore, in the evaluation using other indicators, keywords such as "exacerbation," "pain," and "fever" showed relatively favorable results compared to other keywords. Therefore, these keywords can be considered as candidates for characterizing treatment resistance.
|
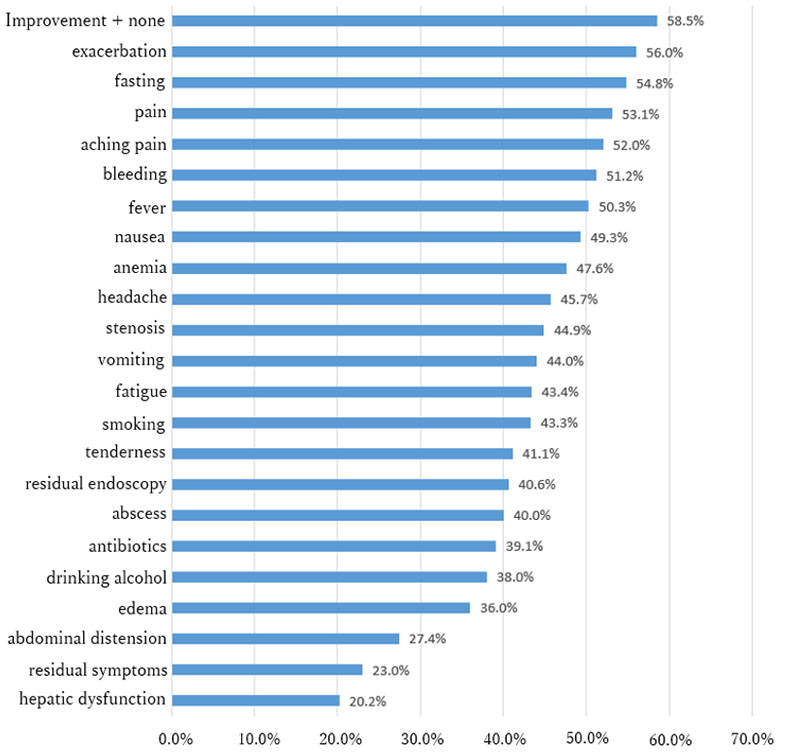
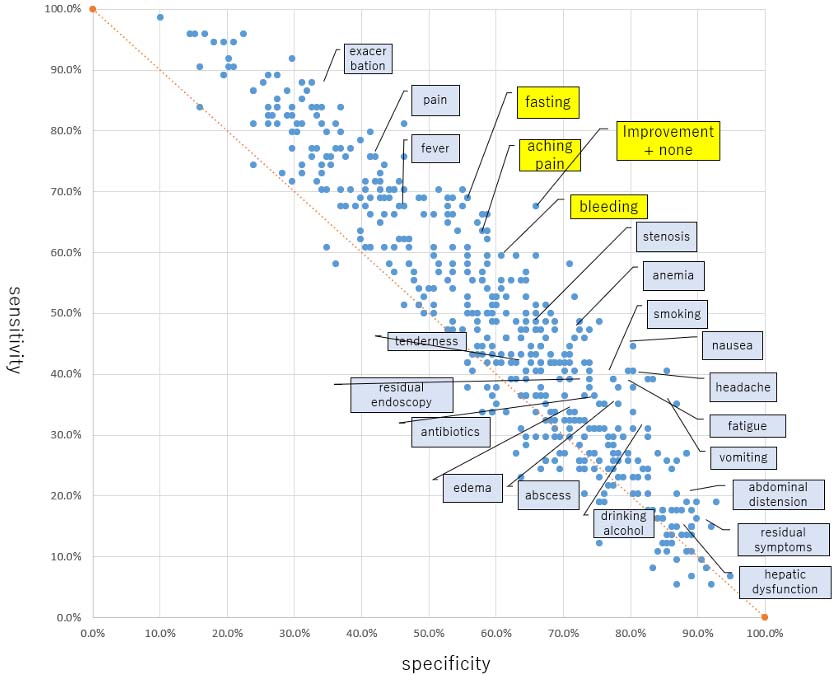
Figure 2: Partial results presented at the academic conference
Future Directions
A key goal of this initial study was determining approaches for future evaluation of methodologies to identify IBD drug resistance. Analysis of keyword frequency shows promise - certain terms demonstrated higher sensitivity and specificity.
Moving forward, NTT DATA's worknclude expanding the lexicon beyond the current keyword sets to improve NLP accuracy. The aim is to utilize expressions extracted from clinician notes and explore combining keywords to enhance precision. Additionally, contextual comprehension techniques like BERT and GPT are viable options worth exploring.
Ultimately, the goals are developing automated determination of treatment resistance and creating digital biomarkers to assist quicker, more efficient delivery of appropriate medications. This will further aid physician decision making and ensure patients receive suitable therapies faster.
NTT DATA's Vision for Pharma
As a collaborator, NTT DATA envisions the future by leveraging foresight-based insights into industries and technologies. With the aim to drive transformation and realize progress together with partners across sectors.
In healthcare, NTT DATA works to achieve patient-centric care through new platforms enabling more personalized experiences. NTT DATA supports pharmaceutical companies' digital shifts, accelerating development while expanding service offerings. Ultimately, NTT DATA's solutions contribute toward seamless healthcare ecosystems centered on the individual.
Related Links
Life Sciences & Pharma | NTT DATA Group
Footnotes
Note 1
Life Data Initiative, a general incorporated association (Certified Producers of Anonymized Medical Data), and NTT DATA (Enterprises Certified for Entrustment with Handling Medical and Related Information and Anonymized Medical Data) collect, anonymize, and provide medical information.
Note 2
Japanese Association for Medical Informatics (JAMI) is the sole member society in Japan affiliated with the International Medical Informatics Association (IMIA).
Note 3
Refers to conditions where standard treatments do not yield sufficient results or where treatment cannot continue due to side effects.
Note 4
Data that functions as an indicator showing a relationship with specific diseases or symptoms obtained through digital devices.
Note 5
Data collected from DPC (Diagnosis Procedure Combination) targeted hospitals for the assessment of the impact of introducing the DPC system. As well as for the future review of the DPC system, including setting scores for each diagnostic group and reviewing diagnostic group classifications.
Note 6
BERT (Bidirectional Encoder Representations from Transformers) is a NLPmodel announced by Google in October 2018. It has since gained significant attention for surpassing the accuracy of conventional models in variousNLP benchmarks.
Note 7
GPT (Generative Pre-Training) is a language model developed by OpenAI that learns from extensive text data. It is noted for generating text with high accuracy that appears as if written by a human. Through specialized domain training, it's capable of classifying input data into various categories.
About ONO Pharmaceutical Co., Ltd.
Ono Pharmaceutical Co., Ltd., headquartered in Osaka, is an R&D-oriented pharmaceutical company committed to creating innovative medicines in specific areas. Ono focuses its research on the oncology, immunology, neurology and specialty research with high medical needs, as priority areas for discovery and development of innovative medicines. For further information, please visit the company's website at https://www.ono-pharma.com/en.
About NTT DATA
NTT DATA provides IT services and business consulting to clients globally. Operating in over 50 countries, the company leverages digital technologies to support organizations undergoing business transformation and help address wider societal challenges. The extensive services offered by NTT DATA span consulting, systems development, and operational support. By working closely with partners, NTT DATA aims to envision future solutions and enact positive change through the smart application of technology.